Abstract
The digital divide is the gap between those with regular; effective access to digital technologies, in particular the Internet, and those without. Researchers have quoted many factors responsible for the digital divide, including: low internet access, low literacy rate, geographical locations, economic conditions, and language barrier. Efforts are being made to provide Internet access by expanding the internet cafés for public use. Communication costs have dropped and many other negative factors have been eliminated or alleviated. One obstacle in bridging the digital divide is the language of the web content. In this paper the authors stress the divide created due to the language barrier. Over 80% of websites are in English despite the fact that less than one in ten people in the world speak that language, which, is one major reason for the digital divide. The majority of Indians, especially those living in rural areas are not proficient in English, which affects both acquisition and dissemination of knowledge by rural communities. This is known as the language barrier, and can be alleviated by machine translation (MT). Machine translation (MT) is the use of computer software to translate text or speech from one natural language into another. Translation of English to Indian languages will provide language-independent interface to the knowledge world and include those excluded from the Web world due to their insufficient understanding of English. This paper is a study of machine translation methods and the various Indian Machine Translation Systems (MTS). In the future, the authors' will focus on developing a MTS to convert the national language (Hindi) to the local language (Dogri) to overcome the language barrier and hence narrowing the Digital Divide.
Keywords: Digital Divide, Machine Translation, Lexicon, Morphology
Introduction
n the past few years, Internet usage worldwide has soared. The Internet has evolved to be the gateway for almost all information that circulates around the world. People have started using the Internet not only for entertainment purposes, but also for knowledge, culture, business, and socialization, making way for a period of great economic prosperity and development in the beginning of the 21st century for almost every nation on the planet. The impact of the Internet on world economic prosperity in the last ten years is so evident that the current economic cycle has been labeled as the "Internet Economy." The widespread use of the Internet has created a divide between those who have access to it and those who do not. One formidable obstacle to the diffusion of Information and Communication Technology (ICT) is language. There is a self-perpetuating cultural hegemony associated with ICTs (Keniston, 2002). By the year 2000, only 20% of all Web sites in the world were in languages other than English, and most of these were in Japanese, German, French, Spanish, Portuguese, and Chinese. But in the larger regions of Africa, India, and south Asia, less than ten percent of people are English-literate while the rest, more than two billion, speak languages that are sparsely represented on the Web. Because of the language barrier the majority of people in these regions have little use for computers. Those who do not use computers have little means to drive market demands for computer applications in their language. National excellence in the millennium will be determined by the extent to which the Information Technology can deliver its potential in local languages. In a country like India, it is crucial to the growth of society and to bridging the Digital Divide that communication overcome the language barrier.
Linguistic Scenario in India
People who lack access to or are unable to utilize these tools, will eventually be unable to function in this increasingly information-based society. | India is a democratic country with a population of over 1 billion. There are about 1650 dialects spoken by different communities. Linguistic-based division into states ensures the use of the official language of that state in governance and education. There are 22 constitutionally approved languages, which are officially used in different states. There are 10 Indic scripts. All of these languages are well developed and rich in content. They have similar scripts and grammars. The alphabetic order is also similar. Some languages use common script, especially Devanagari. Hindi written in the Devanagri script is the official language of the union Government. English is also used for government notifications and communications. India's average literacy level is 65.4 percent (Census 2001). Less than 5 percent of people can either read or write English. Over 95 percent of the population is normally deprived of the benefits of English-based Information Technology[3].As of March 2008; there were 3.3 million active rural Internet users in India. Given the high levels of literacy in rural India and very low levels of English-speaking population, the survey (conducted jointly by IMRB 'Indian Market Research Bureau' and IAMAI 'Internet and Mobile Association of India') made a clear case for content and applications in local languages in order to ensure higher and faster adoption of the Internet in rural India.
Machine Translation
Machine translation (MT) is the use of computer software to translate text or speech from one natural language into another. Like translation done by humans, MT does not simply involve substituting words in one language for another, but the application of complex linguistic knowledge: morphology (how words are built from smaller units of meaning), syntax (grammar), semantics (meaning), and understanding of concepts such as ambiguity. The translation process may be stated as:
- Decoding the meaning of the source text and
- Re-encoding this meaning in the target language.
To decode the meaning of the source text in its entirety, the translator must interpret and analyze all the features of the text. This process requires in-depth knowledge of the grammar, semantics, syntax etc of the source language and the same in-depth knowledge is required for re-encoding the meaning in the target language. In general, a machine translation system contains a source language morphological analyzer, a source language parser, translator, a target language morphological analyzer, a target language parser, and several lexical dictionaries. The source language morphological analyzer analyzes a source language word and provides morphological information. The source language parser is a syntax analyzer that analyzes the source language sentences. A translator is used to translate a source language word into the target language. The target language morphological analyzer works as a generator and generates appropriate target language words for given grammatical information. Also the target language parser works as a composer and composes suitable target language sentences. An MT system needs a minimum of three dictionaries such as the source language dictionary, the bilingual dictionary and the target language dictionary. The source language morphological analyzer needs a source language dictionary for morphological analysis. A bilingual dictionary is used by the translator to translate the source language into the target language; and the target language Morphological generator uses the target language dictionary to generate target language words.
Machine Translation (MT) Methods
Machine Translation is an important sub-discipline of the wider field of artificial intelligence (AI). Some approaches to machine translation are:
- Direct Translation:
is the oldest approach to MT. It is the first generation MTS. In this technique; the source language text is not analyzed structurally beyond morphology. The translation is based on large dictionaries and word-by-word translation with some simple grammatical adjustments e.g. of the word order and morphology. A direct translation system is designed for a specific source and target language pair. The lexicon is conceived of as the repository of word-specific information. These systems depend on well developed dictionaries, morphological analysis, and text processing software. Systran is an example of a direct translation system.
- Rule-Based Machine Translation:
Rule-based machine translation is based on a rich repository of linguistic rules and bilingual dictionaries for each language pair. These complex rule sets are used to transfer the grammatical structure of the source language into the target language. The Interlingua approach and transfer-based MT are types of rule based MT.
- The Interlingua approach:
In an Interlingua-based MT approach, translation is done via an intermediary (semantic) representation of the source language (SL) text. Interlingua is supposed to be a language-independent representation from which translations can be generated to different target languages. The Interlingua approach assumes that it is possible to convert source texts into representations common to more than one language. From such Interlingua representations texts are generated into other languages. Translation is thus in two stages: from the source language to the Interlingua (IL) and from the IL to the target language. The Interlingua approach requires an analyzer for each source language and a generator for each target language. Analysis of source text requires a deep semantic analysis that requires extensive word knowledge
- Transfer Approach
is based on the idea of Interlingua uses contrastive knowledge of two languages. It works in three stages: Analysis, Transfer and Generation. Source language (SL) text is first converted to an abstract SL representation or intermediate representation, which is then changed to target language (TL) representation and finally TL text is produced. In Interlingua-based MT this intermediate representation must be independent of the languages in question, whereas in transfer-based MT, it has some dependence on the language pair involved. It is simpler than the Interlingua approach, but it is difficult to handle ambiguities in this approach.
- Corpus-based
approaches to machine translation use corpora of bilingual parallel texts. The idea of using parallel corpora dates back to the early days of machine translation, but it was not used in practice until 1984. These methods partially succeeded to replace traditional rule-based approaches. The main advantage of corpus-based machine translation systems is that they are self-customizing i.e. they can learn the translations of terminology and even stylistic phrasing from previously translated materials. Statistical MT and Example Based MT are Corpus-Based Machine Translation Methods.
- Example-Based MT:
Example-based machine translation systems are trained from bilingual parallel corpora, which contain sentence pairs like the example. Sentence pairs contain sentences in one language with their translations into another. Example translations are used to train such systems.
- Statistics-Based Machine Translation (SMT):
This approach of translation is based on probability distribution. It utilizes statistical translation models; one approach used is the Bayes Theorem i.e. p (e|f) α p (f|e) p (e) where p (f |e) is the probability that the source string is the translation of the target string, and p(e) is the probability of seeing that target language string. Building statistical translation models is a quick process, but the technology relies heavily on existing multilingual corpora. A minimum of 2 million words for a specific domain and even more for general language are required. Statistical machine translation is CPU-intensive and requires an extensive hardware configuration to run translation models for average performance levels. Google translate is an example of SMT.
- Knowledge-based machine translation
(KBMT) follows the linguistic and computational instructions supplied to it by human researchers in linguistics and programming. The texts to be translated have to be presented to the computer in machine-readable form. It is the knowledge base that converts the source representation into an appropriate target representation before synthesizing into the target sentence. The machine translation process may be unidirectional between two languages: for example, the translation is possible only from Russian to English, and not vice versa, in one system; or it may be bidirectional. KBMT systems provide high-quality translations. However, they are quite expensive to produce due to the large amount of knowledge needed to accurately represent sentences in different languages.
- Hybrid methods
are still fundamentally statistics-based, but incorporate higher level abstract syntax rules to arrive at the final translation. Hybrid approaches use a linguistic method to parse the source text, and a non-linguistic method, such as statistical-based or example-based, to assist with finding the proper interpretation. Such hybrids have been explored in the research community, but without any real success.
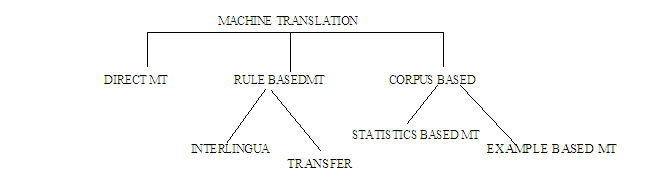
Fig. 1: Diagram showing various MT Approaches
Indian Machine Translation Systems
At present, there are a variety of machine translation systems such as Anusaaraka, Mantra, Angalahindi, etc. Some of them have been discussed below:
Anusaaraka is a popular machine-aided translation system for Indian languages that makes text in one Indian language accessible in another Indian language. This system uses the Paninian Grammar (PG) model for its language analysis. The Anusaaraka project has been developed to translate Punjabi, Bengali, Telugu, Kannada and Marathi languages into Hindi. The approach and the lexicon are general. The output generated is understandable but not grammatically correct. The system has been applied mainly to children's stories.
MANTRA(Machine Assisted Translation Tool) is one of the Web-enabled machine translation systems, which translates the English text into Hindi in a specified domain of personal administration, specifically gazette notifications, office orders, office memorandums, and circulars. It uses Tree Adjoining Grammar (TAG) for Parsing and Generation and a bottom-up parsing algorithm to speed up the parser and online word addition and grammar updating facilities.
Angalahindi is web-based English to Hindi machine-aided translation system. It is a version of Anglabharati. It is specifically designed for translating English to Indian languages. English is a SVO (subject-verb-object) language while Indian languages are SOV (subject-object-verb) and have a relatively free word order. Instead of designing translators for English to each Indian language, Anglabharti uses a pseudo-interlingua approach. It analyses English only once and creates an intermediate structure called PLIL (Pseudo Lingua for Indian Languages).The PLIL structure is then converted to each Indian language through a process of text-generation. It is used for translation from English to all Indian languages.
UNL-based English-Hindi machine translation system: The Universal Networking Language (UNL) is an international project of the United Nations University, with an aim to create an Interlingua for all major human languages. IIT Bombay is the Indian participant in UNL, and it is working on MT systems between English, Hindi and Marathi using the UNL formalism. This uses an Interlingua approach--the source language is converted into UNL using an 'enconverter', and then converted into the target language using a 'deconverter'.
Shiva and Shakti machine translation: The Shiva and Shakti are the two Machine Translation systems from English to Hindi and have been developed jointly by Carnegie Mellon University USA, International Institute of Information Technology, Hyderabad, and and Indian Institute of Science, Bangalore, India. The system Shiva is an Example-based and the system Shakti is working for three target languages like Hindi, Marathi and Telgu. Shakti MTS has been designed to produce machine translation systems for new languages rapidly. The Shakti system combines rule-based approach with statistical approach whereas Shiva is an Example-Based machine translation system.
Hindi to Punjabi machine translation system:
The Hindi to Punjabi Machine translation System was developed by Goyal and Lehal (2010) at Punjabi University Patiala in the year 2009. This system is based on direct word-for-word translation approach. This system consists of modules like pre-processing, word-for-word translation using a Hindi-Punjabi lexicon, morphological analysis, word sense disambiguation, transliteration, and post-processing. The system has reported 95% accuracy.
Conclusion
The Internet may become the main medium of communication in the future.As the internet becomes the increasing vital tool in our society and technology provides increasing options to citizens to conduct their daily activities online such as learning, shopping, payment of bills, registration of licensees etc.; people who lack access to or are unable to utilize these tools, are at a growing disadvantage and will eventually be unable to function in this increasingly information-based society. Therefore there is an urgent need to bridge the digital divide and provide digital opportunities to those excluded from the Web. Machine Translation enables localization of information, and is very important in a linguistically diverse country like India. Machine translation has enabled communication in the users' native language; thus removing the language barrier among people and reducing the digital divide. Many languages have been translated, yet a lot many need translation.
Future Scope
A number of Machine Translation systems between Indian and non-Indian languages have already been developed; but there is still no Machine Translation system for Hindi to Dogri (the regional language of Jammu). In the future. the authors will focus on developing such a MT system so that Dogri can be made a part of the Web.
References
[1] Keniston Kenneth. (2004). "Introduction: The Four Digital Divides". In K. Keniston and D. Kumar (Eds.) IT Experience in India. Delhi: Sage Publishers
[2] Mário Rodrigo Canazza, "Global Effort on Bridging the Digital Divide and the Role of ICT Standardization", in Proc IEEE Conf on Innovations for Digital Inclusions on Aug 31-Sept 1, 2009, page(s): 1-7
[3] Om Vikas, "Multilingualism for Cultural Diversity and Universal Access in Cyberspace: an Asian Perspective", UNESCO, 6-7 May 2005.
[4] www.wikipedia.org
[5] http://iamai.in/PRelease_detail.aspx?nid=1754&NMonth=1&NYear=2009
[6] N. Balakrishnan, "Information and communication technologies and the digital divide in the Third World countries",
Current Science, Vol. 81, No. 8, 25 October, 2001
[7] Mark Warschauer, "Technology and Social Inclusion: Rethinking the Digital Divide" (Cambridge, MA: MIT Press, 2003), 274 pp.
[8] Pandey et al, "From Digital Divide to Digital Opportunity", Proceedings of IEEE Region10 Conference, 19-21 Nov. 2008, page(s): 1 -6.
[9] Budditha Hettige et al, "Web-based English-Sinhala translator in action", in Proc IEEE Conf on Information and Automation Sustainability, on 12-14 Dec 2008 on pages 80-85.
[10] Vishal Goyal and Gurpreet Singh Lehal, "Web Based Hindi to PunjabiMachine Translation System", Journal of Emerging Technologies in Web Intelligence, Vol. 2, No. 2, May 2010, pg(s):148-151.
[11] Anusaraka: A Device to Overcome the Language Barrier, V.N. Narayana, Ph.D. thesis, Dept. of CSE, I.I.T. Kanpur, 1994.
[12] Bharati, A., R. Moona, P. Reddy, B. Sankar and D.M. Sharma et al., 2003. Machine translation: The Shakti approach. Proceedings of the 19th International Conference on Natural Language Processing, Dec. 2003, India, pp: 1-7.
|